Discover how causal AI helped identifying complex root cause chains in battery manufacturing.
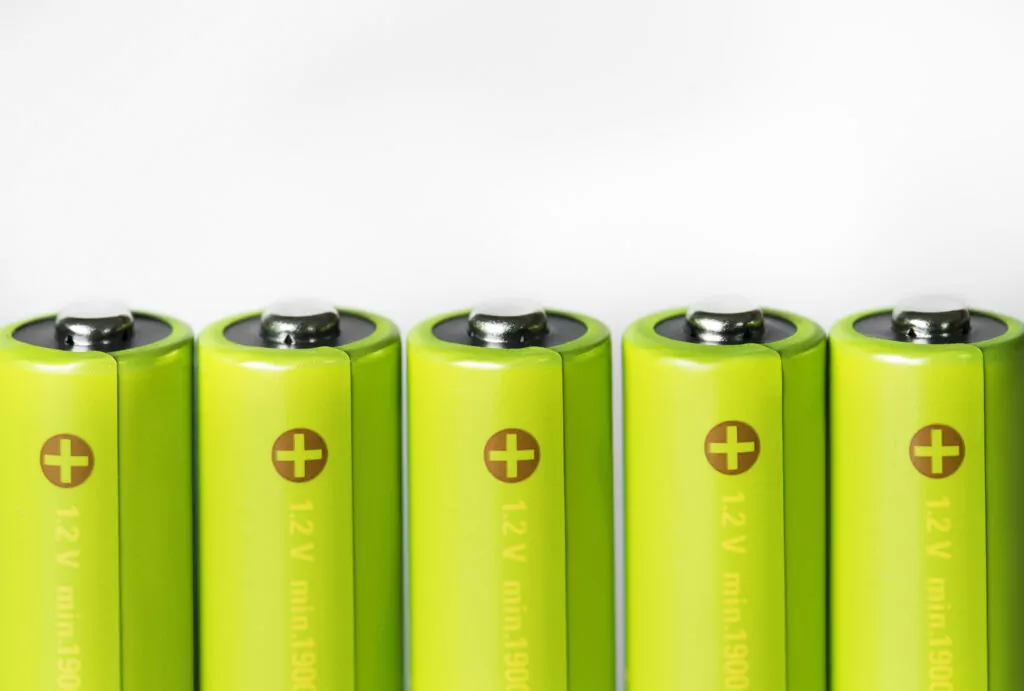
Industry
Electronics
Location
Europe
Product
Analyst
Battery manufacturing is a highly complex and precise process where even minor deviations can have a significant impact on product quality. One critical quality measurement in battery manufacturing is cell voltage, which directly affects the performance and reliability of batteries. In this case study, we describe how the implementation of causal Artificial Intelligence (AI) played a significant role in identifying root cause chains behind variations in cell voltage over time. A battery manufacturer was facing severe quality variation in one production line. Specifically, cell voltage stability frequently fell below acceptable limits. Traditional statistical process control (SPC) was unable to pinpoint the root causes of these voltage fluctuations, leading to increased scrap and rework costs.
Traditional methods of root cause analysis often fall short and fail to deliver conclusive results in complex manufacturing environments. This is particularly true for the identification of root cause chains, where multiple process parameters generate a chain reaction throughout a manufacturing process.
Industry Expert
We collected a dataset that ranged from process parameters and raw material properties to product quality attributes, with a special emphasis on cell voltage stability. To address the intricate issue of identifying root causes behind voltage variations, we utilized the causal AI capabilities of the EthonAI Analyst software.
Alongside the dataset, we incorporated specific domain expertise into the Analyst software. This expertise included detailed knowledge about the production flow, such as the process sequence and the parameters measured at each production step. As a result, the Analyst software was able to find the root cause chain, which ultimately enabled it to track down the root cause of declining cell voltage. Unlike conventional machine learning techniques that often stop at identifying patterns in data, our advanced graph models reveal the underlying factors causing those patterns. This is particularly important in complex manufacturing systems where understanding causal relationships leads to more effective quality improvement actions.
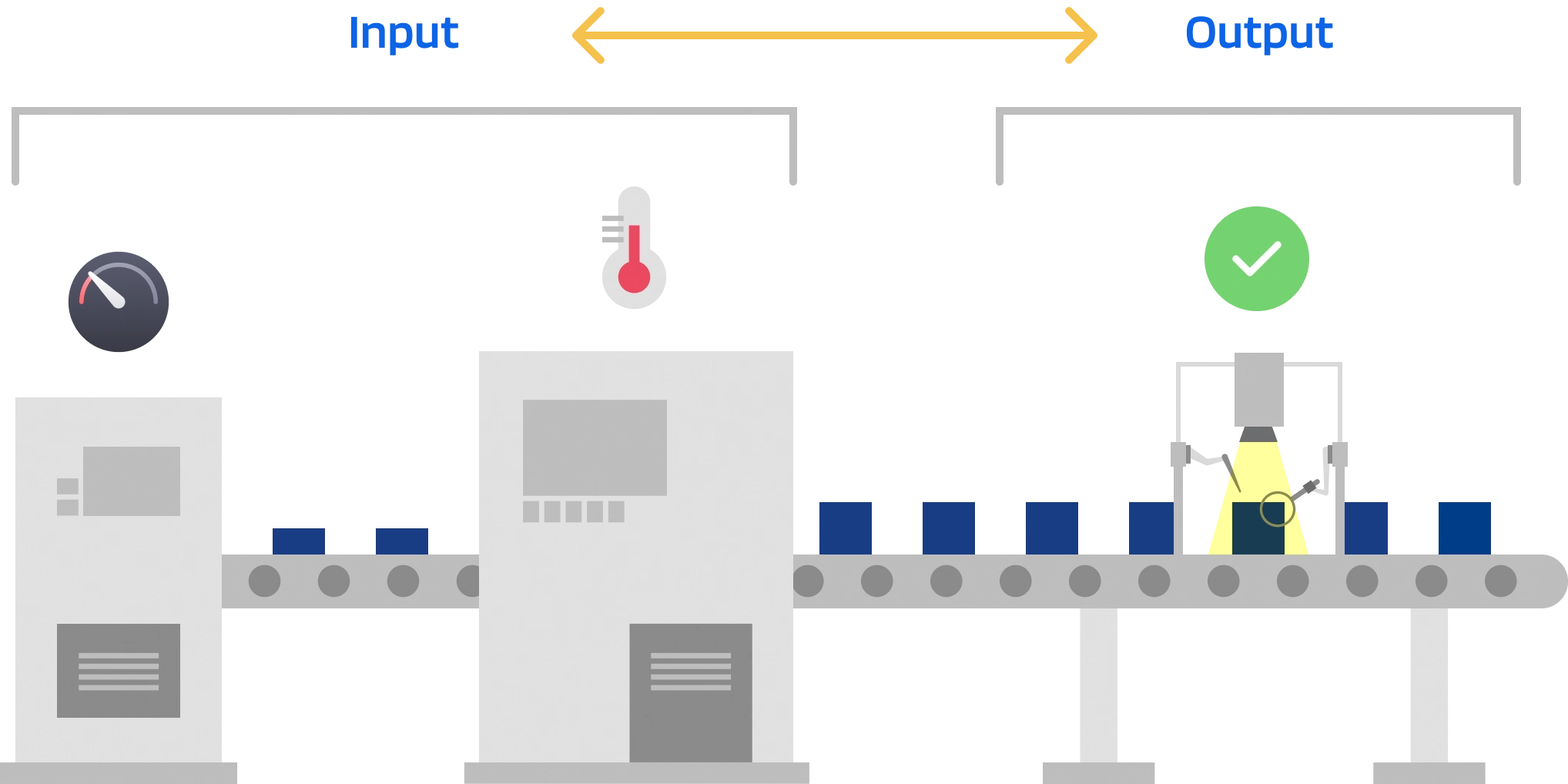
Industry Expert
The Analyst software identified two key root causes—welding current and electrolyte concentration—that had a direct impact on cell voltage stability. Unlike traditional methods, which typically overlook upstream factors, our model successfully back-tracked to the root causes influencing these direct effects. Specifically, the software revealed calibration issues in one of the assembly machines affecting welding current. Similarly, variations in electrolyte concentration were traced back to the electrolyte supplier. These upstream root causes had been overlooked in traditional analyses but were crucial in understanding the effects on the cell voltage.
This case study demonstrates the distinct advantages of employing causal AI in complex manufacturing systems. The causal AI capabilities of the EthonAI Analyst enable the back-tracking of root causes through the incorporation of domain knowledge and by considering the flow through a factory. This approach helped pinpointing how cell voltage was affected by upstream root causes, which could not be discovered by traditional correlation analysis. The insights presented in this case study show how to remedy current inefficiencies, and also provide a robust framework for future quality management initiatives.
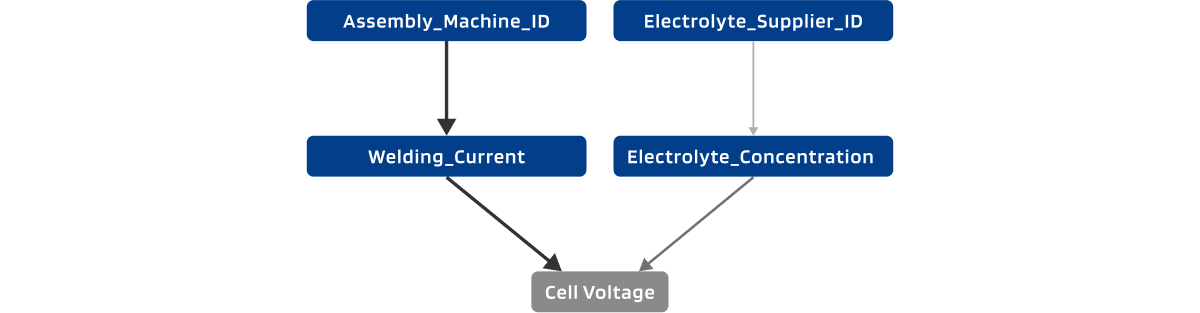
Impact
Causal AI tackles tough quality problems
Uncover Interactions
Unstable cell voltage was traced to a combination of machine miscalibration and one supplier's electrolyte concentrations.
Upstream Fixes
Yield losses that previously appeared unavoidable, and could only be caught late in the process, can now be fixed much earlier.
Domain Knowledge
Being able to easily inform the algorithm of domain expertise was crucial. Experts don't need to write any code, they simply provide hints about possible links.