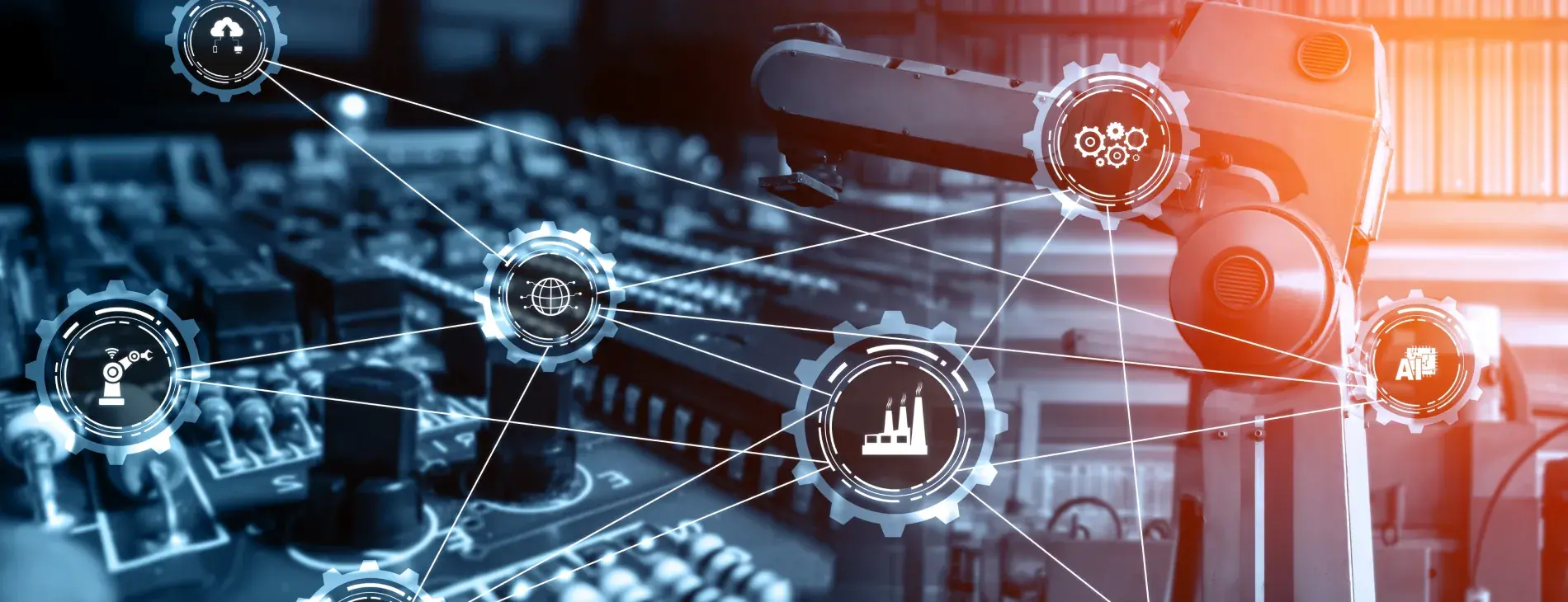
Blog
Introducing the Manufacturing Analytics System
Modern factories generate substantial amounts of data, but frequently, it is not effectively utilized. This article explores how a new software category — the Manufacturing Analytics System — helps manufacturers turn their data into valuable insights for productivity improvement.
The need for a new software category in the manufacturing industry
The manufacturing industry is currently undergoing a significant transformation. Growing process complexity, changing macroeconomic trends, and rapid technological advancement make it increasingly urgent and difficult to achieve operational excellence.
In response to these changes, manufacturers need to find new ways to improve their productivity. Recent technological advances are a great opportunity to support manufacturers in this endeavor. Over the past years, there has been considerable progress in manufacturing data collection, which has been spurred by the development of IIoT sensor technologies, standardized network protocols, and cloud-based storage and computation. However, the mere collection of data does not automatically lead to increased productivity in factories. Often, this data is not effectively utilized to drive improvements.
Despite significant digitization efforts, the effective use of analytical tools in today’s factories remains limited. For many manufacturers, the situation can be best described as being data-rich but information-poor. According to IBM, approximately 90% of all sensor data is never analyzed. This is disappointing, because the lack of operational insights is currently considered as a key obstacle that manufacturers need to overcome.
What has led us to this situation? In essence, 21st century factories are still managed with 20th century tools. Data is stored in disjoint sources, software is fragmented and verticalized, there is no standardization across sites and teams. Consequently, key employees such as operations managers, process experts, and data scientists are bogged down by the extensive efforts required in aggregating and cleaning data. It is evident that we need unification of analytical standards and workflows to leverage existing data much more effectively.
The answer to these challenges is a new category of software: The Manufacturing Analytics System (MAS). A MAS creates a common context across disparate data sources, analyzes data with the latest AI techniques, and makes the results accessible in a suite of interoperating applications. The applications in a MAS are tailored for the different people involved in achieving operational excellence. A MAS serves as an intermediary between data and users, provides deeper insights faster, enables new types of automation, and streamlines the looping of decisions back to the factories. It makes employees considerably more effective and improves operational KPIs sustainably.
Under the hood of the Manufacturing Analytics System
Manufacturing Analytics Systems are designed to serve end-users across all levels of operational excellence, ranging from factory floor personnel to upper management. A MAS is structured around three layers that transform diverse manufacturing data into valuable insights for productivity improvement:
- an Application Layer,
- a Model Layer,
- and a Context Layer.
The Application Layer contains user-facing software tools that enable productivity improvement. These tools are built on top of a Model Layer, which houses specialized AI models that are designed to generate insights or decisions from manufacturing data. The Context Layer is responsible for gathering data from a wide range of origins and channeling it into the Model Layer for processing. Each of the layers is described in more detail below.
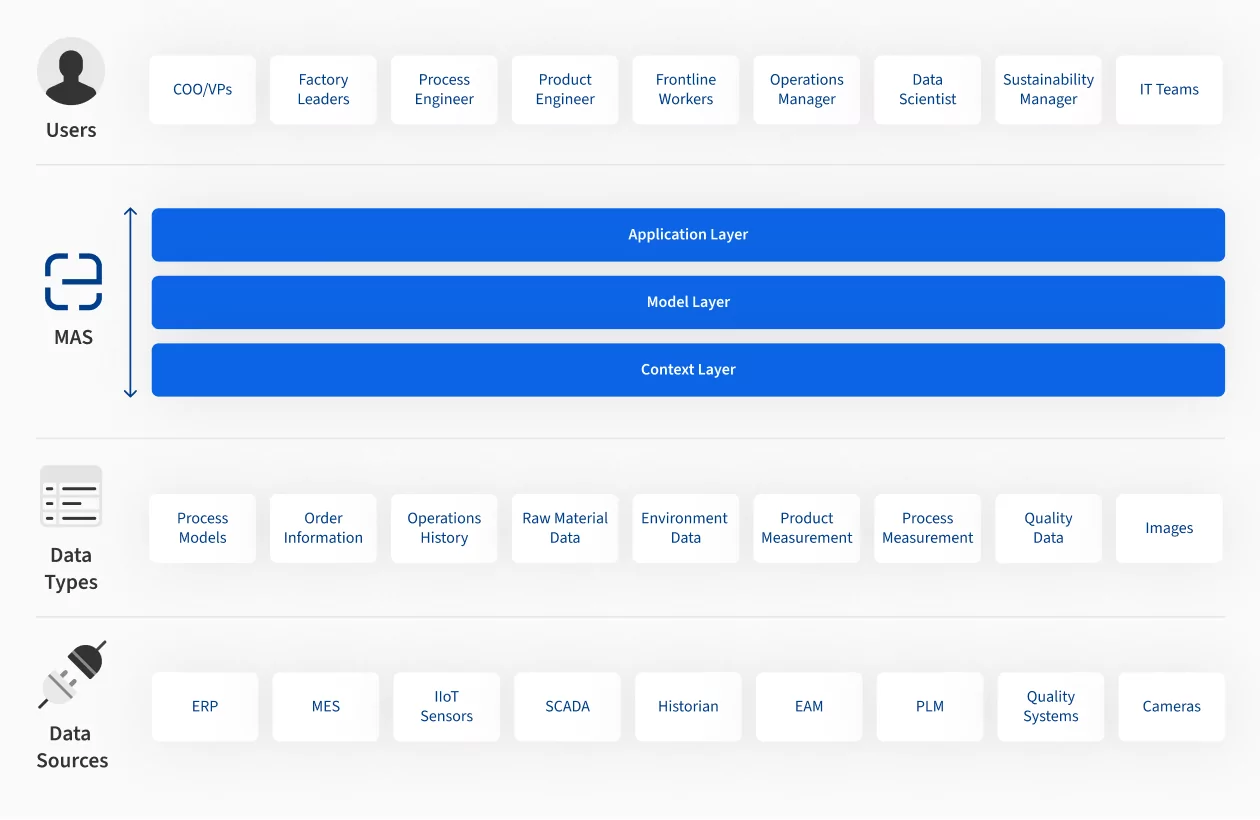
Context Layer
The Context Layer serves as the foundation of a MAS. It prepares and organizes the data for its use in the Model Layer. This layer does not duplicate existing databases. Instead, it stores only relevant data, merged from different sources, in a unified and aggregated format. It provides the crucial link across disparate data sources. This is achieved by mapping or creating common identifiers like timestamps, part IDs, and batch IDs. The context and connections in this layer enable comprehensive analysis across different datasets.
Model Layer
The Model Layer comprises advanced AI models for complex data analysis in manufacturing. Unlike common data platforms that use off-the-shelf algorithms (e.g., Random Forests, GBM, ResNets, etc.), this layer involves tailored models specifically designed for manufacturing tasks (e.g., root cause analysis, visual inspection, material flow analysis, etc.). Tailored models in the Model Layer enable a MAS to effectively address challenges where generic approaches fail.
Examples include EthonAI’s graph algorithms for root cause analysis, LLMs trained on manufacturing queries, and specialized computer vision models for quality inspection.
Application Layer
The Application Layer contains the user-facing applications that leverage the data processed by the underlying layers. It is a no-code/low-code environment where users engage with the relevant information in tools that are custom-built for manufacturing workflows. This layer is designed to be intuitive for a large set of user personas and to directly integrate into their workflows.
Examples include EthonAI’s Analyst, Inspector, Observer, Tracker, and Miner software.
How will the Manufacturing Analytics System change the industry?
Over the past five years, industry leaders have significantly improved their data acquisition capabilities. Over the next five years, we expect the mid-market segment will catch up. This opens the door to unprecedented productivity levels in the industry. But to really capitalize on their data assets, manufacturers need stronger and widespread analytics. Manufacturing Analytics Systems will quickly become essential for this purpose.
A MAS offers manufacturers advanced analytical capabilities and user-centric applications to improve operational decision-making. It equips manufacturers with the right set of tools to operate as effectively as lighthouse factories. The MAS is not just a technological advancement; it represents a paradigm shift in manufacturing. It commoditizes access to advanced analytics for the wealth of manufacturing data and enables widespread improvement of operations.
EthonAI is building the applications and infrastructure to lead this transformation. Explore the Use Cases on our website to discover how EthonAI’s MAS is already used by industry leaders to cut production losses and achieve operational excellence today.